Study presents a conceptual framework for enhanced aquafeed design
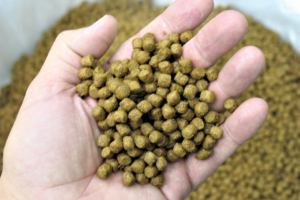
Significant efforts continue to source alternative, lower-cost ingredients for aquafeeds. Research has focused on the replacement of fishmeal with other ingredients, such as plant byproducts, algae (micro and macro), insects, land animal byproducts and single-cell proteins (including bacteria and yeast).
Alternative protein sources need to meet a number of criteria if they are to be deemed commercially viable. These criteria include (i) nutritionally adequate (e.g., digestible and does not significantly impair fish growth performance or physiological and health status); (ii) palatable to the fish; (iii) scalable to commercial levels; (iv) physically stable; (v) easily handled and stored; (vi) crucially, have lower environmental and lifecycle impacts. Finally, any feed must meet commercial realities, namely profitability, if they are to successfully be used by feed manufacturers. Given the complex criteria that feeds are required to meet, there is a gap in the tools available for feed formulation that balance economic and environmental efficiencies.
This article – adapted and summarized from the original publication (Cooney, R. et al. 2021. Designing environmentally efficient aquafeeds through the use of multi-criteria decision support tools. Current Opinion in Environmental Science & Health, Vol. 23) – proposes a multi-criteria decision support tool that leverages machine-learning [ML, the study of computer algorithms that can improve automatically through experience and by the use of data. ML algorithms build a model based on sample data, known as “training data,” to make predictions or decisions without being explicitly programmed to do so] techniques and presents a conceptual framework to interrogate different datasets to identify more efficient feed formulations faster.
Towards sustainable feeds: Replacing trial and error approaches
Advanced computational and statistical modelling through machine learning have been applied to areas such as medicine, renewable energies and wastewater treatment to optimize the design or operation of various products and processes. Such a step does not preclude the need for in vivo or in vitro experimental validation but enables a more targeted approach to the initial selection of product features. This has been demonstrated by efforts during the COVID-19 pandemic, where the role of machine-learning models led to the rapid development of new vaccines. Machine-learning models were used to investigate viral infection pathways, model biomolecule responses and aid in the identification of favorable response patterns within the training data.
Machine-learning capabilities have been previously used in aquaculture for disease detection, water quality monitoring, on-site management, farm site selection, risk assessment and in feeding regimes. Machine-learning applications in aquaculture feed formulation (and indeed other food sectors reliant on complimentary feed) can provide a bridge between disciplines such as industrial ecology, biology, environmental engineering and nutrition to arrive at precise aquafeeds.
In one conceptual example, the use of neural networks [an artificial network, composed of artificial neurons or nodes, which may be used for predictive modeling, adaptive control and applications where they can be trained via a dataset. Self-learning resulting from experience can occur within networks, which can derive conclusions from a complex and seemingly unrelated set of information] could allow for the use of meta-datasets and literature to help design options for a least-cost feed formulation, which is species-specific, using low environmental impact ingredients, while shortening the supply chain and optimizing fish growth (Fig. 1). Such a tool could leverage large datasets to identify patterns and generate new formulations that meet the specific needs of the fish. This multi-criteria approach has been investigated in the literature using linear programming and has been termed multi-objective formulation.
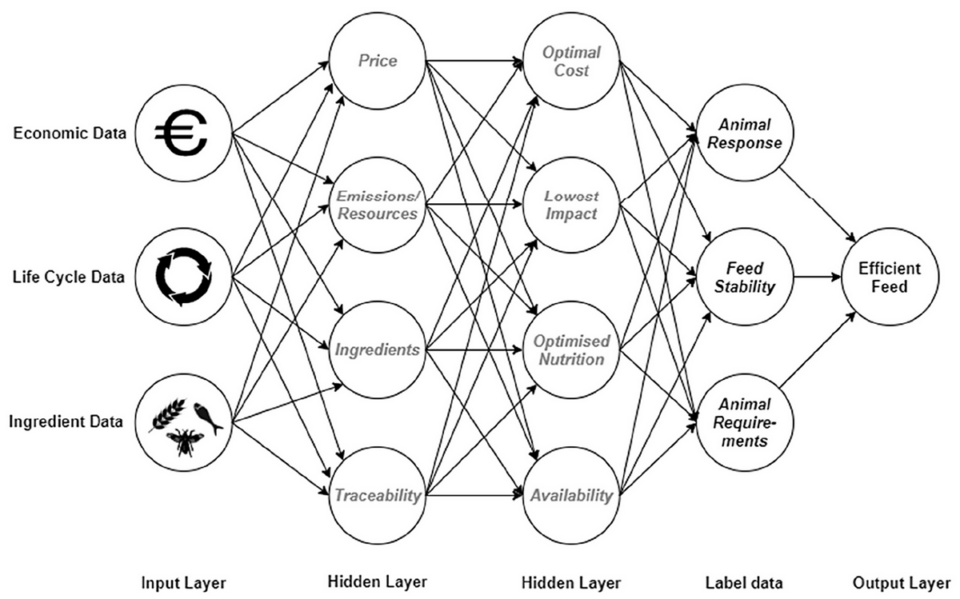
The use of machine learning as part of this formulation allows for a bolt-on approach to these established methods and may present new possibilities with regards to automation and process efficiency while aligning with emerging policies. By iterating new product prototypes through “virtual” trial and error experiments that also simulate fish response, the feed development paradigm can be revolutionized. Emerging trends of explainable machine learning can further be used to interrogate meaningful connections within feed formulations and associated fish response for enhanced insight.
Data availability
The links between aquafeed ingredients, nutritional profile and environmental impact have been included in several major feed ingredient studies and databases. In lieu of experimental data, public and proprietary datasets can be used to develop machine learning models. For example, nutritional datasets and expected impacts on the farmed animals can be sourced from online open-access feed information databases such as Feedipedia, IAFFD and others. These systems collate feed data and act as repositories for information on established, historical and emerging feed ingredients. They include statistics on variability, which can be used for predictive modelling or uncertainty analysis.
Various life cycle databases can be integrated with the aforementioned nutritional datasets, and economic data on feed ingredients could be sourced from online databases, price indexes or through industry partnerships. These industry partnerships can be difficult to form due to intellectual property rights and commercial sensitivities; however, the knowledge they generate can significantly advance topics and open new avenues of research. Some examples are the involvement of the aquafeed company Sparos through the GAIN project, AllerAqua as part of the FutureEUAqua and iFishIENCI projects.
Feed to farm
Farmed aquatic animal nutrient emissions (e.g., faecal matter and ammonia) from modeled feeds can be approximated within a machine-learning model. Nutritional models have been used in the approximation of fish metabolic wastes in lifecycle assessment [LCA, a methodology for assessing environmental impacts associated with all the stages of the lifecycle of a commercial product, process, or service] of finfish aquaculture. The linking between formulation and use can further expand the scope of machine learning models in aquaculture, allowing developers to estimate the impacts across the production chain (raw material, production and use). Such data can also be aligned with data from long-term studies of discharges to water from aquaculture facilities. This builds on emerging trends in machine learning to combine real and synthetic or generated data to improve model training.
Extracting relevant data for each of the ingredients from the nutritional, economic and lifecycle datasets can allow for the first step in the development of a broader feed design tool. The next step involves transforming the data into a form amenable for use in specific machine learning algorithms.
Using various computational programming approaches can uncover hidden patterns in complex environmental, economic and nutritional datasets that can be used to generate efficient feed formulations faster. This can improve the efficiency of trial-and-error approaches (which it should be said are backed up by significant expertise) and in turn, generate more optimized feeds that can be tested and embedded into sustainable feed supply chains. This switch from a model-centric to a data-centric approach means that there is no restriction on the type of data that is to be considered and enables nonlinear relationships to be interrogated.
Other considerations
The development of a multi-criteria decision support tool is a step to optimize economic costs, maintain the nutritional value and nutrient digestibility while minimizing the environmental impacts (Fig. 2). Assessing these factors rapidly in a simulated manner can enable feed manufacturers and researchers to disrupt the feed development process, optimize the design of feeds before the trial stage, and target the design of feeds with specific priorities in mind.
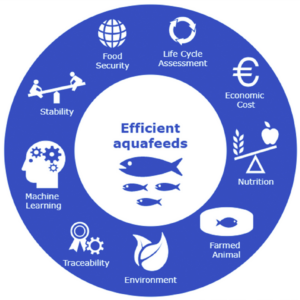
This tool should be grounded in lifecycle thinking. As such, it is necessary to expand the boundaries of the tool and connect it with other previously proposed machine learning-based applications and tools. Such an expansion would allow for the accounting of direct, indirect and induced impacts, which feed production and use, can have on the lifecycle of aquaculture products. Efficient feed formulation can only increase the sustainability of aquaculture so far.
Poor feed management at the farm level can result in nutritional deficiencies in the stock and/or excessive nutrient loading to the water system. This can introduce or exacerbate the spread of disease within the farmed fish stock, leading to increased feeding requirements and higher environmental burdens (e.g., due to chemical treatments to ameliorate health concerns, eutrophication processes from excess feed deposition). This farm-level data can be connected with explainable machine learning tools that allow stakeholders to interrogate both the generated feed formulations, the underlying reasons that make these formulations the optimal choice and optimal management practices.
A finalized or commercial version of the approach discussed here could include the use of blockchain to increase traceability and security through the supply chain while meeting consumer or market demands. Blockchain has in recent years become a popular means of ensuring the providence of ingredients and products. Its inclusion and use in aquafeeds can help to bolster consumer confidence and perceptions in the face of negative publicity, such as those surrounding supply chains of fishmeal.
With more food products and production systems using blockchain as a means of increasing traceability, its inclusion into the aquafeed production chain can position aquaculture as a prime example of a resource-efficient and traceable food production system. This combination of efficient feed and advanced traceability of the final farmed product could appeal to environmentally conscious consumers while also meeting the requirements of emerging food security and traceability legislation, such as EU Regulation 2018/775 and the European Union Farm to Fork strategy.
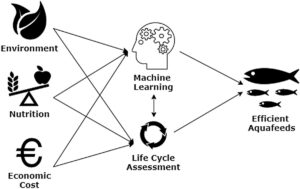
The linking of LCA, nutritional data, production processes, traceability and economics has significant potential as a disruptive innovation to enhancing the sustainability of farmed finfish and shrimp. The adopting of a data-centric rather than model-centric approach introduces a paradigm shift where the large volumes of (training) data available allow a machine learning model to learn the pertinent (nonlinear) relationships and mappings between given inputs and outputs. This approach has enormous advantages in terms of simplifying deployments and allowing models to be applied to a wide variety of feed formulations (since only the data changes).
Semi-automated machine learning frameworks such as Lale could be used to simplify further model development. In using a data-centric approach, there are no restrictions to data that can be considered. The application of machine learning to the questions posed by sustainable feed formulation opens up the field to a technology that has made enormous strides across multiple industries over the past decade.
Perspectives
This article outlines and presents the benefits for the interlinking of economic, nutritional and environmental impact datasets to develop an innovative framework for feed formulation design for farmed aquatic animals using machine learning. There are numerous datasets and databases available to develop the structure and tools necessary to implement such a system. The benefits that this avenue of research has to offer can increase the sustainability of aquaculture and strengthen its role in feeding the world.
Now that you've reached the end of the article ...
… please consider supporting GSA’s mission to advance responsible seafood practices through education, advocacy and third-party assurances. The Advocate aims to document the evolution of responsible seafood practices and share the expansive knowledge of our vast network of contributors.
By becoming a Global Seafood Alliance member, you’re ensuring that all of the pre-competitive work we do through member benefits, resources and events can continue. Individual membership costs just $50 a year.
Not a GSA member? Join us.
Authors
-
Ronan Cooney
Corresponding author and Ph.D. candidate
School of Engineering, National University of Ireland Galway, H91 HX31, Ireland; and
Ryan Institute, National University of Ireland Galway, H91 TK33, Ireland -
Alex H.L. Wan, Ph.D.
Ryan Institute, National University of Ireland Galway, H91 TK33, Ireland; and
Aquaculture and Nutrition Research Group (ANRU), Carna Research Station, National University of Ireland Galway, Carna, Co. Galway, H91 V8Y1, Ireland -
Fearghal O'Donncha, Ph.D.
IBM Research Europe – Dublin, Damastown Ind. Park Mulhuddart, Dublin 15, Ireland
-
Eoghan Clifford, Ph.D.
School of Engineering, National University of Ireland Galway, H91 HX31, Ireland; and
Ryan Institute, National University of Ireland Galway, H91 TK33, Ireland
Tagged With
Related Posts
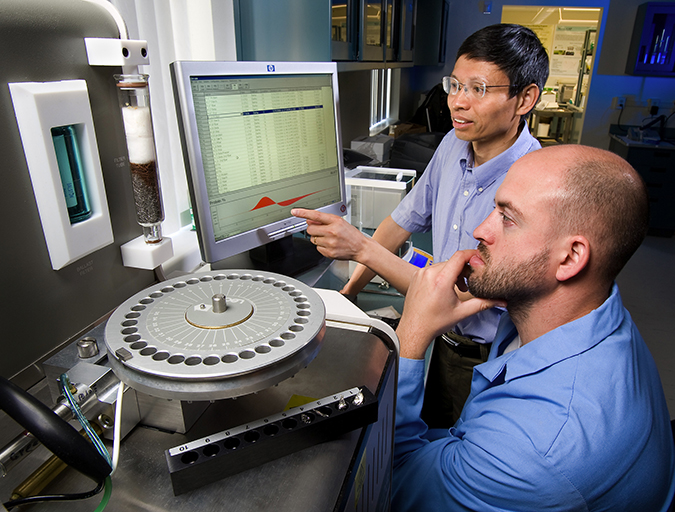
Aquafeeds
Alternative feed ingredient universe to convene at F3 meeting
What started out as a simple yet ambitious contest to drive innovation in the aquafeed sector has evolved into a fully global competition – and collaboration – amongst ingredient suppliers and feed manufacturers.
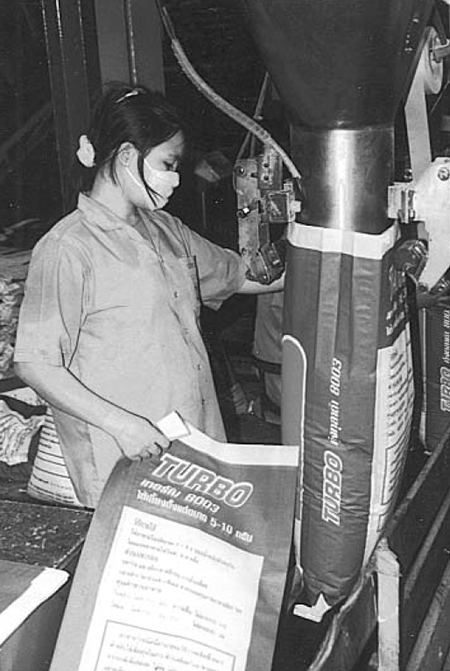
Aquafeeds
Ecofeeds and the coming ecotechnology in aquaculture
Clearly, there will be no single solution to our needs, but rather a combination of biotechnological and ecotechnological solutions.
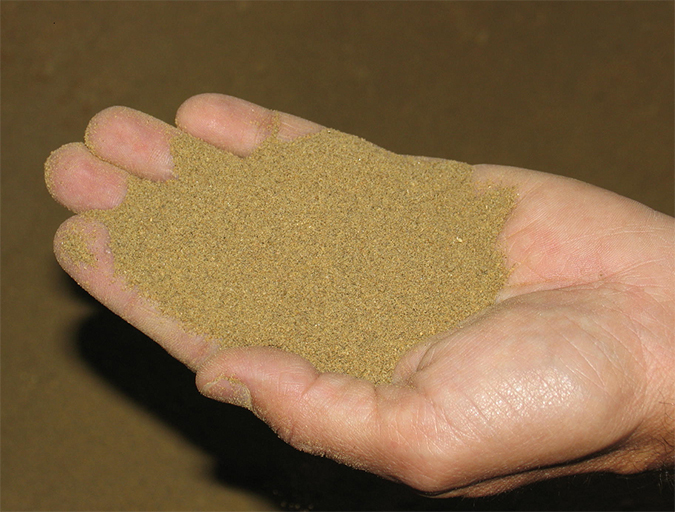
Aquafeeds
Mixing Cost Center is at the heart of feed manufacturing
Ingredients that are properly proportioned and mixed for an adequate mixing time are very important to assure the manufacturing of aquafeeds that meet the standards for the shrimp species and life stage for which the formula was designed.
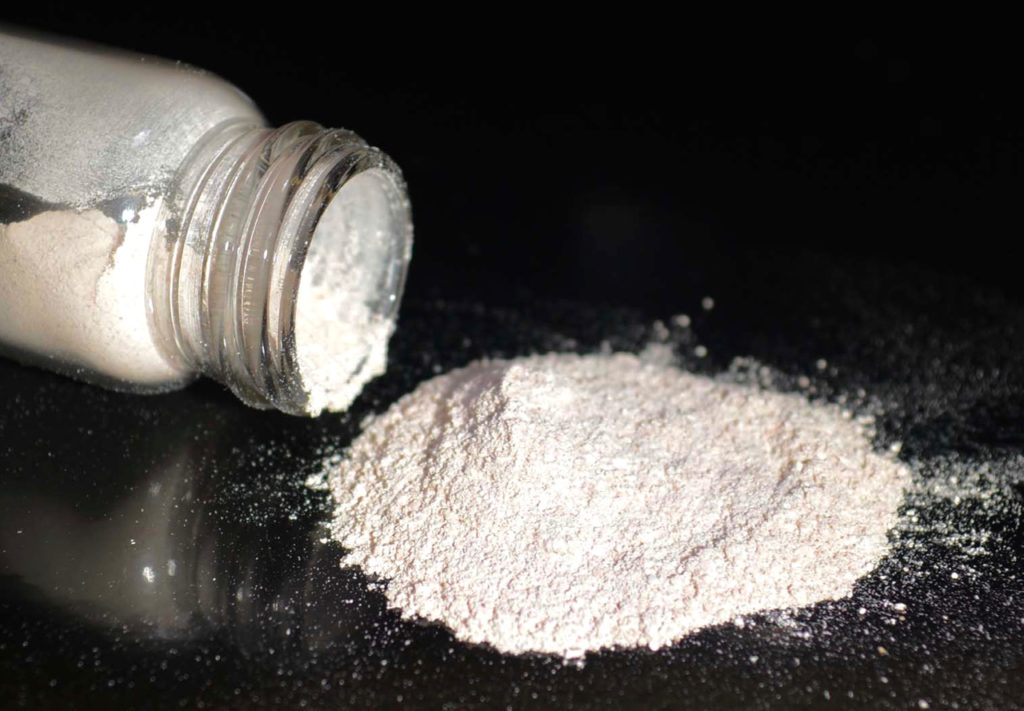
Aquafeeds
A new nutrient for aquaculture, from microbes that consume carbon waste
Biotechnology firm NovoNutrients aims to produce a line of nutraceutical aquafeed additives as well as a bulk feed ingredient that can supplement fishmeal. Its process includes feeding carbon dioxide from industrial gas to a “microbial consortium” starring hydrogen-oxidizing bacteria.