Novel approach combines available predictive tools and state-of-the-art machine learning methods, bridging a gap between traditional and modern techniques in fish age prediction

The European hake (Merluccius merluccius) is a significant demersal finfish in the marine ecosystems of the North Atlantic and Mediterranean seas. The Cape Breton Gorge on the Atlantic coast of North America divides two hake stocks, the northern and the southern, with the southern stock highly overexploited. The European hake is also one of the most valued and overfished species in Western European fisheries. Its high commercial value has resulted in population declines over the previous 20 to 40 years as a consequence of fishing pressure from commercial fisheries in the Eastern Mediterranean. Due to the significance of age estimation for stock assessment and fisheries management, age identification remains essential for the management and conservation of the species and the production of accurate stock assessment models.
Fish age determination is paramount for assessing growth rates, population dynamics, future population trends and the overall ecological state. Additionally, age and growth assessments of commercial fish species are essential inputs in age-structured assessment models, which are used to evaluate the level and impact of exploitation on fish stocks. Scales, otoliths (ear bones) and vertebrae are used in traditional age categorization methods, but these methods are often time-consuming, labor-intensive, prone to human error and susceptible to environmental influences. Despite their shortcomings, otoliths comprise an essential tool for fisheries assessment today.
The otolith reading approach is an internationally accepted technique for determining the age of M. merluccius. The interpretation of the otolith ring formation employed for the age estimation of M. merluccius exhibits an unusual complexity, posing several challenges that include the description of the otolith’s core, the presence of false annual rings and difficulty in ring border interpretation.
These challenges result in otolith readings that are often open to interpretation, leading to confusion and disagreement among scientists. Despite European hake’s high commercial and significant ecological value, concerns surrounding the interpretation of its age have yet to be resolved.
This article – summarized from the original publication (Klaoudatos, D. et al. 2024. From Data to Insight: Machine Learning Approaches for Fish Age Prediction in European Hake. J. Mar. Sci. Eng. 2024, 12(9), 1466) – discusses a study to (i) to identify otolith morphometric factors that significantly affect age estimation for European hake; (ii) formulate a simple, reliable, and easy to use model for the age estimation of European hake based on these previous factors; and (iii) with the application of machine learning (ML; a field in artificial intelligence to develop and study statistical algorithms that can learn from data and generalize to unseen data, and perform tasks without explicit instructions) methodology, further develop a framework to predict the age of European hake using limited otolith morphometric characteristics.
Study setup
A total of 150 M. merluccius individuals were collected from the Central Aegean Sea (Eastern Mediterranean) using a commercial trawl vessel and the fish otoliths were collected and processed. Age reading was independently performed by three readers.
Then, a multivariate methodology identified the morphometric factors that significantly affect age estimation, and easy-to-use equations using limited morphological otolith characteristics with a high degree of accuracy were produced as a practical tool for fisheries management. A second tool using machine learning (ML) algorithms produced a highly accurate ML model with the ability to further predict European hake’s age using limited otolith morphometric characteristics.
Both tools are important for assessing fish population dynamics, managing sustainable fishing practices, and ensuring the long-term health of marine ecosystems. The models could be implemented by collecting fish otolith samples, measuring limited morphometric features using imaging techniques, and inputting these measurements into the machine learning model.

For detailed information on the collection, processing, imaging, morphometry and age classification of the hake otoliths, statistical analyses, machine learning and modeling and other techniques used, refer to the original publication.
Results and discussion
Our research provides a valuable preliminary exploration into the application of otolith morphometric features for age estimation in European hake. This study introduces an innovative approach that offers a precise and efficient alternative to traditional methods, which are often labor-intensive and prone to human error. The advancements presented here hold potential for improved population assessments and more effective management strategies for this commercially significant species.
Additionally, the findings offer a foundation for future exploration of machine learning applications in other marine species, potentially leading to the development of generalized age prediction models. This aligns with contemporary trends in fisheries science, where advanced computational methods are increasingly applied to address complex ecological and biological challenges.

Our results provided two practical tools for fisheries management by estimating fish age from otolith morphometric characteristics. The first tool was an easy-to-use equation with a high degree of accuracy, using only otolith weight, form factor and aspect ratio to predict European hake’s age. The second tool was a highly accurate ML model with the ability to further predict European hake’s age using the same inputs (otolith weight, form factor, and aspect ratio). Both tools (refer to the original publication) are important for assessing fish population dynamics, managing sustainable fishing practices and ensuring the long-term health of marine ecosystems.
The models could be implemented by collecting fish otolith samples, measuring limited morphometric features using imaging techniques and inputting these measurements into the machine learning model. Both model outputs will allow researchers and fisheries managers to obtain rapid and reliable age estimates without the need for labor-intensive traditional methods. By integrating these models into routine fisheries assessment workflows, stakeholders could make more informed decisions about fish stock assessments and conservation strategies.
The starting point of the stock assessment often consists of research that focuses on the life-history traits of fish species. Consequently, otolith reading procedures that are validated through international standards and standardized could be an effective tool to advance our understanding of those issues that fishery biologists continue to vigorously address. Otolith reading-based M. merluccius aging research can yield extremely valuable information since it is widely acknowledged that accurate data on the age structure of the catch is crucial for stock evaluation. Fish age can be inferred from otolith morphological and morphometric properties, demonstrated to be age-related.

Since fewer fish may be age-analyzed as a result, monitoring programs are forced to rely more heavily on growth-at-age models in order to ascertain the age composition of fish populations. This emphasizes the necessity for automated tools that otolith experts could use to enable a more efficient study in their facilities. Additional research and widespread implementation of these methods could be advantageous for each of these domains. By assessing stock conditions along with offering reliable species classification, this approach could contribute to the goal of sustainable fisheries. Moreover, the use of image recognition algorithms could lessen the need for laborious manual measurements by non-invasively evaluating features like length and scale radius from photographs.
Artificial neural networks can predict age in Greenland halibut using otolith images
Fish age determination using otolith imaging has proven to be an important tool for fisheries management, offering reliable data leading to the estimation of critical trends in fish biology and population dynamics. Fisheries programs have been obliged to restrict the quantity of fish specimens analyzed and rely on model estimations to assess the age composition of fish populations due to the high expenses associated with data collection and age interpretation. This highlights the need for automated techniques to determine fish age. Our results indicated that the application of ML algorithms may assist in fish age recognition and become an asset for experts in age reading.
The significance of this research transcends the European hake, offering a foundation for the broader application of machine learning in fisheries science. This approach may facilitate the development of generalized age prediction models that are applicable across various species. As fisheries management increasingly relies on data-driven strategies, the integration of advanced computational techniques, as exemplified in this study, is poised to play a critical role in addressing the complex challenges associated with sustainable fishery practices and biodiversity conservation.
The advent of digital technology, image analysis systems and shape analysis methodologies has significantly boosted the importance of studies on otolith morphology. The specific benefits include a low cost and relatively high efficiency, making the study of otolith morphometry crucial for stock identification and fisheries management. Instead of requiring laborious manual measurements, image recognition algorithms could be used to non-invasively evaluate features from images, such as length and scale radius. Additionally, using real-time data, ML models have the potential to predict growth patterns.
Perspectives
This study identified the main contributing otolith morphometric factors that significantly affect the age estimation for European hake, namely, otolith weight, form factor and aspect ratio. Three easy-to-use equations with a high degree of accuracy were further produced and are readily applicable for most stakeholders. With the application of ML methodology, a framework was further developed for the prediction of European hake’s age from otolith morphometrics.
The integration of imaging technology, employed to assess otolith morphology, and statistical analyses offers a powerful approach to fish age identification, with high accuracy, a reduction in labor and the enabling of large-scale assessments. Age and growth studies could utilize otolith morphometry in age estimation, resulting in time and cost reductions, while generating reliable data. To guarantee a thorough prediction, an accurate assessment, and overall sustainable stock exploitation, it is also crucial to consider the relationships and interactions between the environmental elements in the aquatic ecosystem.
By the careful selection of datasets and model evaluation, ML could address biases and overcome the drawbacks of traditional approaches that rely on subjective judgments. With age estimation being essential to studies of the stock assessments and population dynamics of the European hake, the present study indicates the potential for accurate age estimation and the expedition of the assessment process, providing researchers and fisheries managers with trustworthy data for conservation and sustainable resource management.
Our study results provide a valuable preliminary exploration into the application of machine learning for the otolith-based aging of European hake. Despite its limitations, the study offers a robust starting point that can significantly contribute to the field and guide subsequent research efforts. Future studies could employ larger sample sizes to validate and extend our findings. The current study lays the groundwork and provides a proof-of-concept, which is crucial for securing funding and resources for larger-scale research.
Now that you've reached the end of the article ...
… please consider supporting GSA’s mission to advance responsible seafood practices through education, advocacy and third-party assurances. The Advocate aims to document the evolution of responsible seafood practices and share the expansive knowledge of our vast network of contributors.
By becoming a Global Seafood Alliance member, you’re ensuring that all of the pre-competitive work we do through member benefits, resources and events can continue. Individual membership costs just $50 a year.
Not a GSA member? Join us.
Author
-
Dr. Dimitris Klaoudatos
Corresponding author
Department of Ichthyology and Aquatic Environment (DIAE), School of Agricultural Sciences, University of Thessaly (UTh), Fytokou Street, 38446 Volos, Greece
Tagged With
Related Posts
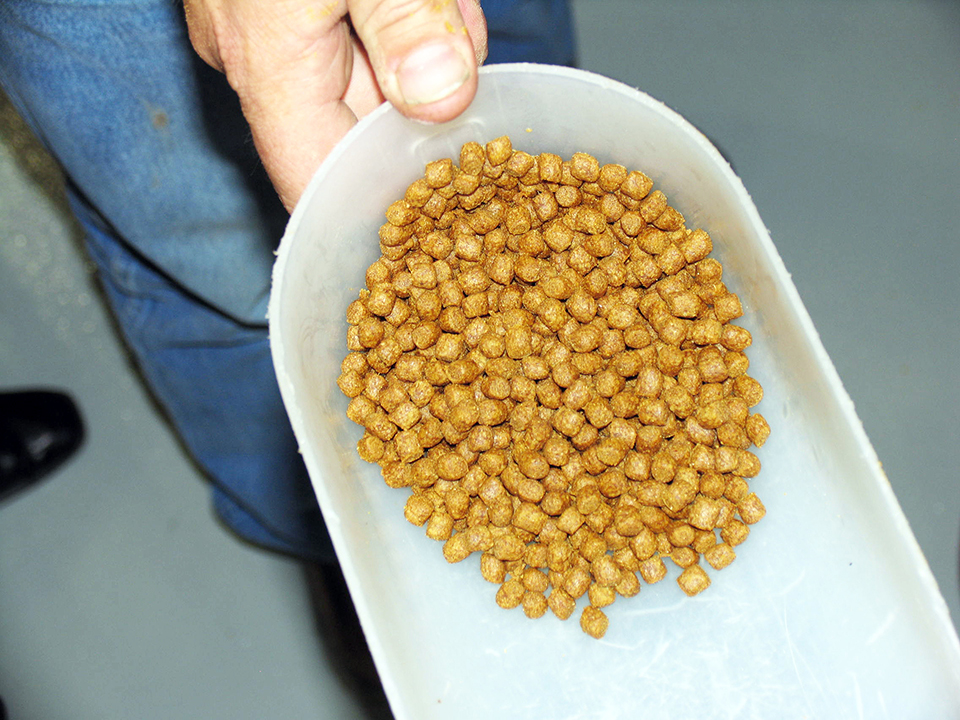
Intelligence
Byproduct utilization for increased profitability, part 5
Fish protein hydrolysates have been used in the food industry for the development of antioxidants, emulsifiers, flavoring agents, anti-bacterial agents and feed. As natural products, they have a growing acceptance, since natural products are preferred by some consumers over industrial products.
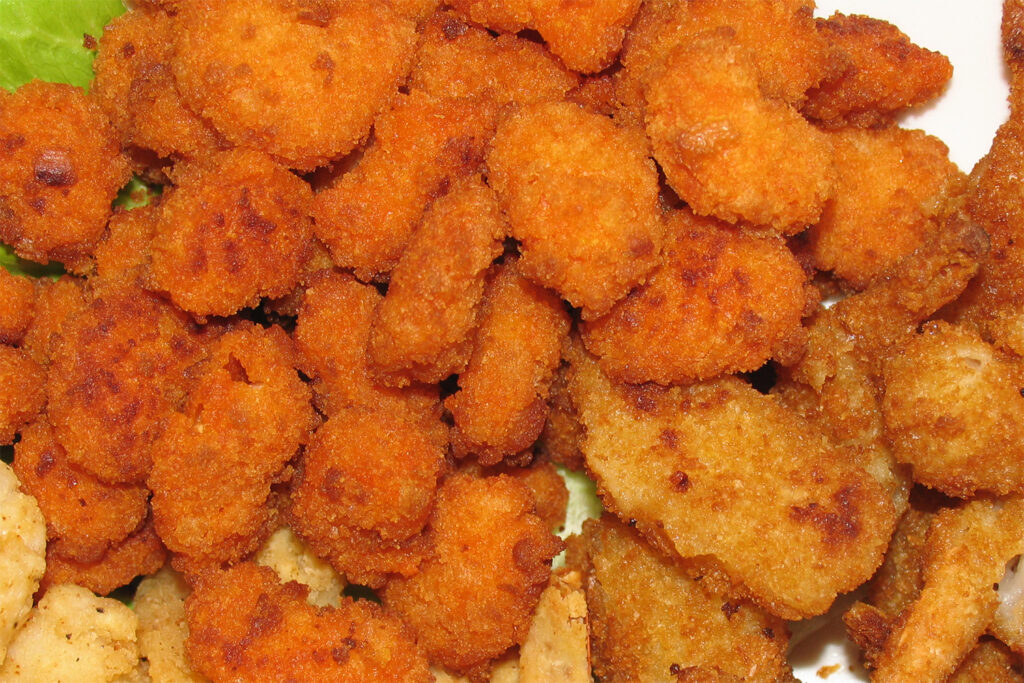
Intelligence
Using DNA multi-marker metabarcoding to authenticate processed seafood
Next Generation Sequencing techniques can support routine food industry activities and official control programs to combat fraudulent practices.
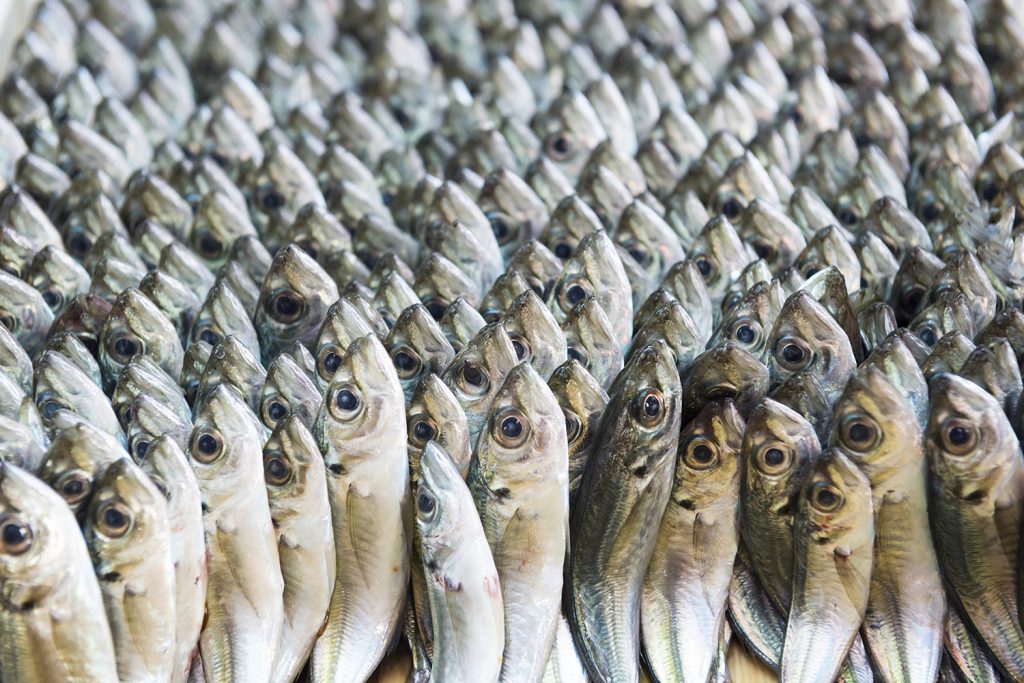
Intelligence
Can handheld DNA testing technology stand up to seafood fraud?
The MasSpec Pen, developed to diagnose tumors, can identify fish species by touching the tip to a sample. But the species database is lacking.
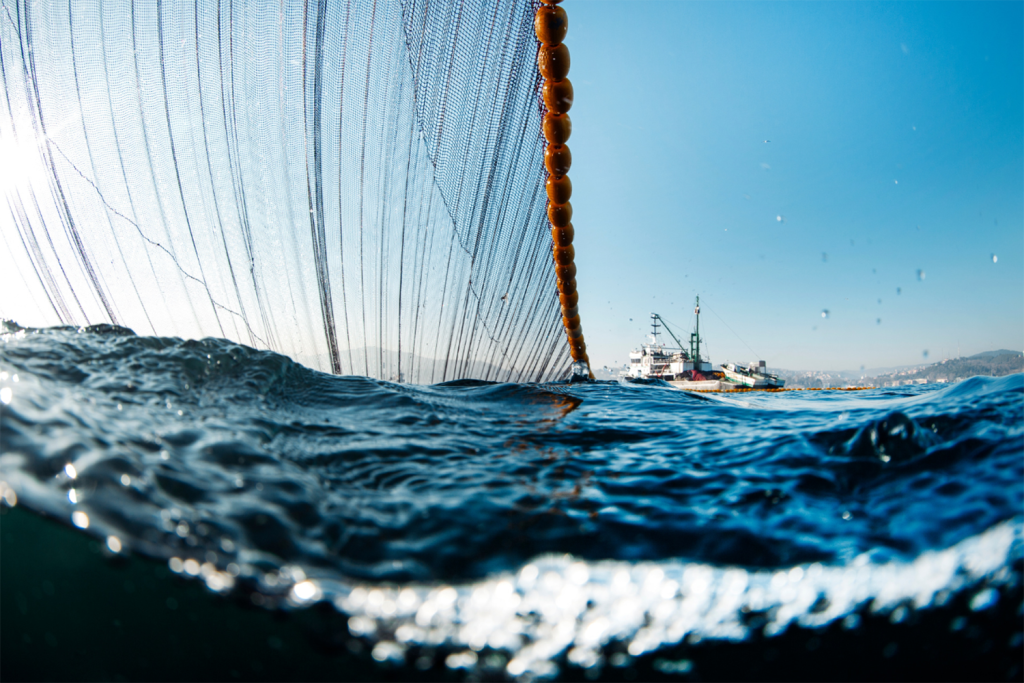
Fisheries
‘They need a good reason to stay’: How one coalition may break a decade of deadlock in the North Atlantic mackerel fishery
GOAL 2022: The North Atlantic Pelagic Advocacy Group, led by Dr. Tom Pickerell, is a finalist for GSA’s inaugural Global Fisheries Innovation Award.