Identification performance scores obtained were sufficiently high to effectively distinguish between the different tuna species evaluated
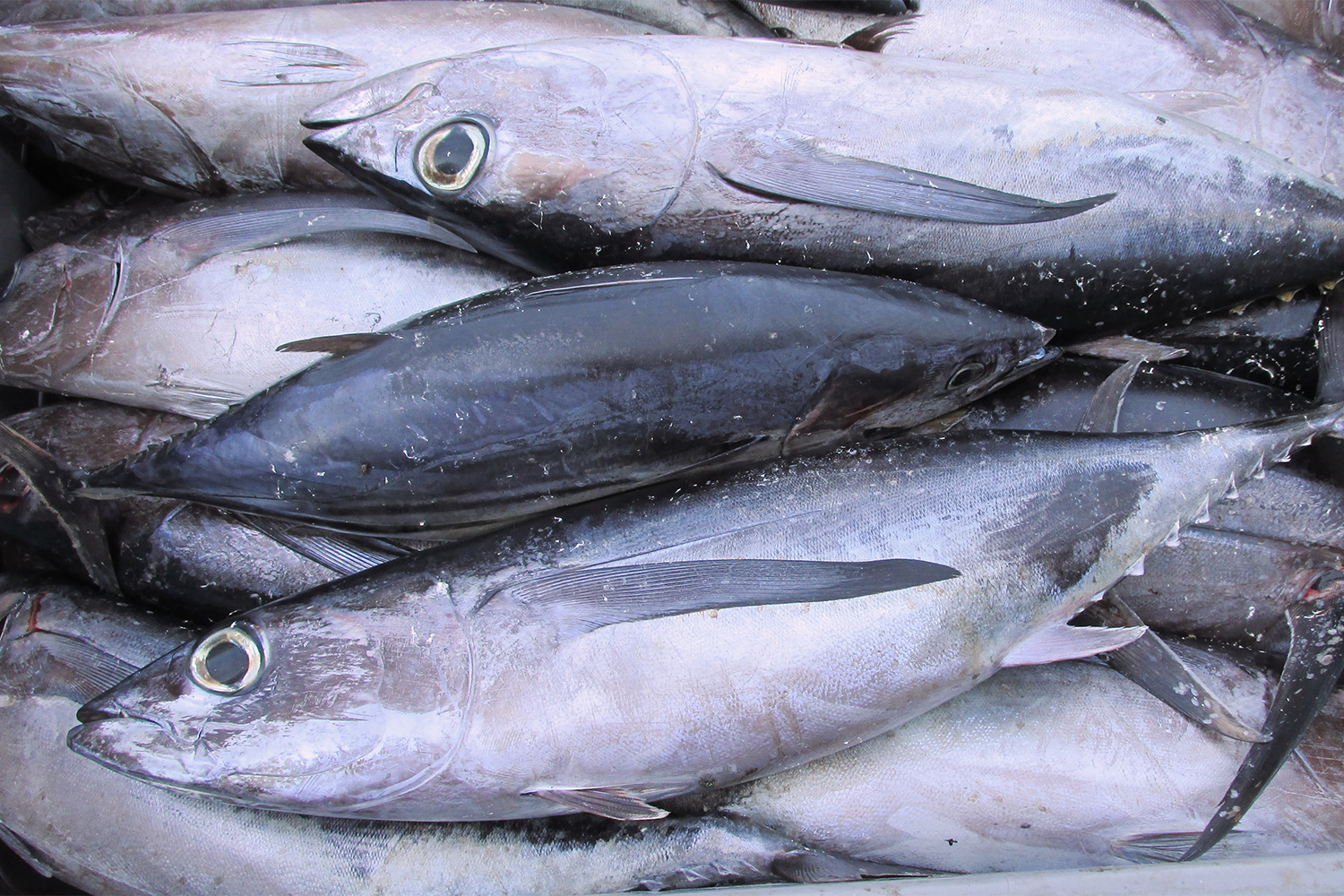
Tunas are important targets for large-scale international industrial marine fisheries around the world. Many important species of tuna have significant values, both economically and nutritionally, and play a significant role in the economic development of many countries. In addition, tunas are among the most widely consumed seafood in the world.
The development of artificial intelligence technology has pushed the gradual development of fisheries management toward automation and informed direction across the globe. Machine learning is at the center of artificial intelligence and is a key technology for realizing automated fish identification. Combined with high-performance computers, machine learning technology can mine high-dimensional features and deep information in data, thereby offering a solution to automated fish identification and fishery monitoring, and introducing the fishery industry into a new era.
As the scale of marine fishing continues to expand, more and more attention has been focused on fish biodiversity. In the future, tuna catches are likely to transform into a new sustainable fishing model with automation and intelligence. Therefore, the automated identification of tuna species will help to protect tuna biodiversity, which is significant for improving the economic efficiency of fishing enterprises, enhancing fishery resource assessment and sustainable management, and ensuring sustainable fishery production in the future.
This article – summarized from the original article (Ou, L. et al. 2023. Automated Identification of Morphological Characteristics of Three Thunnus Species Based on Different Machine Learning Algorithms. Fishes 2023, 8(4), 182) – reports on a study for the automated identification of three tuna species through morphological characteristics based on different machine learning algorithms.
How aging uncertainty of major tuna species impacts the estimation of growth functions
Study setup
This research studied the biological diversity of different tuna species of the same genus and analyzed the identification performance of machine learning for different morphological features. A total of 300 individuals of bigeye tuna (Thunnus obesus), yellowfin tuna (T. albacares) and longfin tuna (T. alalunga) were used as research objects – 100 of each species (Fig. 1) that are among the most important commercial tuna species for global tuna fisheries.
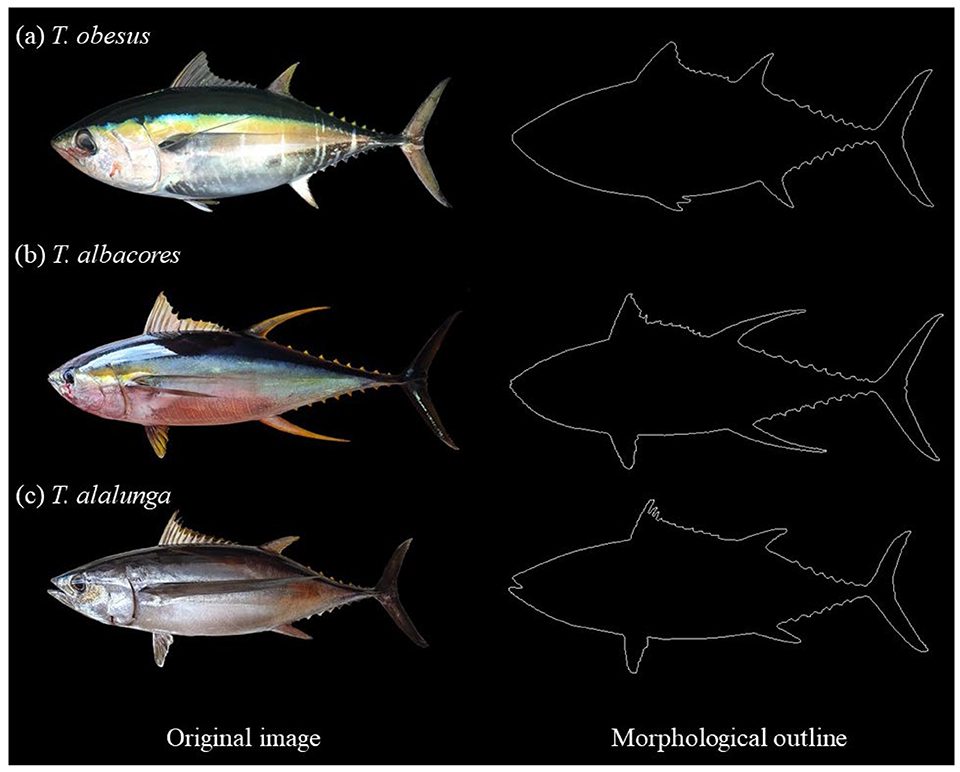
The digital images of tuna were collected by observers on board. Images were acquired using a digital camera and a smartphone. Surveys were conducted in the Central Ocean and Western Pacific Ocean from March 2021 to September 2022. The tunas were aligned horizontally and centered in the images, and the images were processed to give images 400 pixels high × 800 pixels wide, then saved.
For detailed information on the automated identification methods of three tuna species, including obtaining the morphological outline images; data transformations; and the use of different machine learning algorithms on morphological feature data, as well as other analyses, refer to the original publication.
Results and discussion
Morphological characteristics are an important feature commonly used in automated fish analyses. On the one hand, morphological characteristics are determined by the nature of fish. Many fish species can adapt to ecosystem modification because they are naturally plastic in terms of their morphology. On the other hand, morphological characteristics are determined when the fish develops. Morphological characteristics in fish are often associated with development, growth rate, nutrition, and environmental conditions.
Morphological information has significant advantages in fish bioecology, so it has a scientific theoretical basis for the automated analysis of fish morphological characteristics. The analysis of morphological characteristics allows a detailed description of their shape and outline, which can be compared intra- or interspecifically. Morphological characteristics have been used for the automated identification of fish.
A key need for the monitoring of fisheries is comprehensive and reliable fish data. Hence, the automated analyses of fish morphology can facilitate electronic access to information, and the automated identification of tuna would contribute to the precise quantification of the catch, thus supporting the development of modern fishery management.
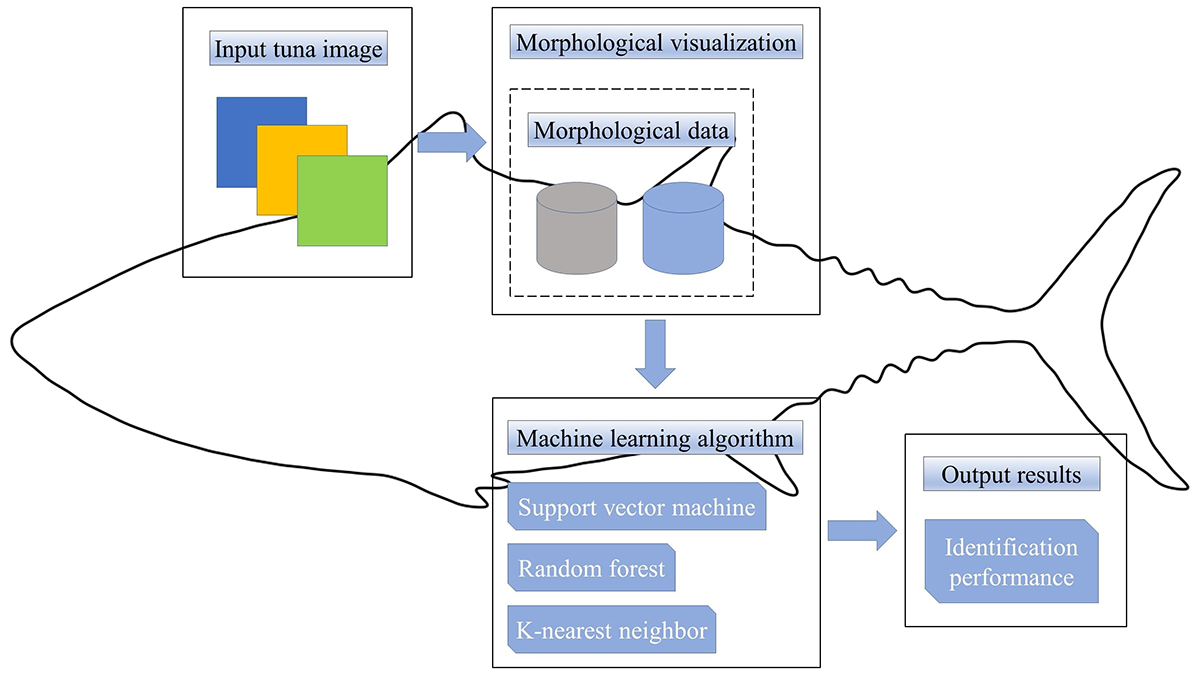
The two morphological characterization methods used in this study could well identify the three Thunnus species. In the morphological reconstruction of the Elliptic Fourier Transform (EFT, a technique that permits comprehensive quantification of outline shapes) method, the change process of tuna morphology was from abstract to concrete. In the morphological visualization analysis of the CNN [convolutional neural network, a class of artificial neural network (a method in artificial intelligence that teaches computers to process data in a way that is inspired by the human brain) most commonly applied to analyze visual imagery] method, the change process of tuna morphology was from concrete to abstract. By comparing the visual images of the EFT and CNN methods, we confirmed that CNN could also qualitatively analyze the morphological diversity of tunas.
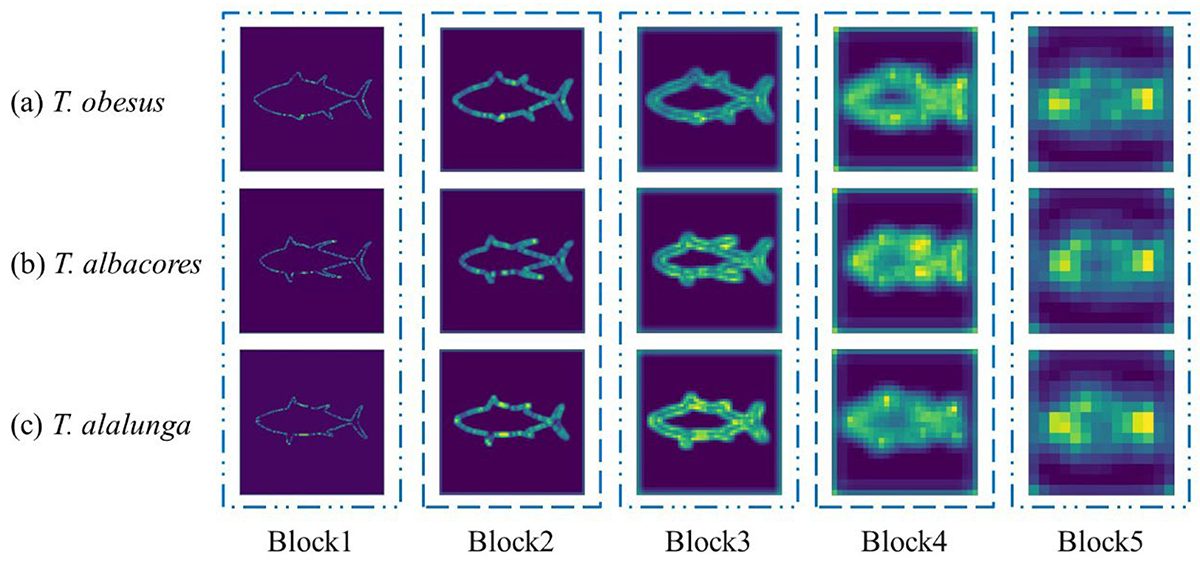
The data of the two different morphological features were analyzed using principal component analysis (PCA, a popular technique for analyzing large datasets containing a high number of dimensions/features per observation, increasing the interpretability of data while preserving the maximum amount of information, and enabling the visualization of multidimensional data). The cumulative contribution rate of the two morphological features in the top ten principal components was more than 80 percent. It was further found that each principal component of morphological characteristic data showed significant interspecific differences among Thunnus species. It was preliminarily verified that the obtained data of different morphological features were adequate, and they provided a good database for subsequent automated identification.
Overall, the identification accuracies for T. obesus, T. albacares and T. alalunga were 90 percent, 90 percent and 85 percent, respectively. The difference among species was mainly reflected in the fins. The identification performance of each species of tuna using different morphological characteristics and different machine learning algorithms revealed significant differences. However, each tuna species has essential diversity characteristics, and therefore the identification performance score was sufficiently high to effectively distinguish different tuna.
In addition, similar to previous studies, deep features combined with machine learning algorithms directly extracted all the information of the original fish images for research and consequently obtained good identification results. This further verifies that deep features combined with a machine learning algorithm can be applied to fish species identification. Future studies may better apply CNN to fish biology research and fish diversity analysis by identifying tuna of the same genus and different species based on their morphological outlines.
Perspectives
This study involved the use of an automated method for the identification of three Thunnus species through morphological features based on different machine-learning algorithms. The method uses EFT features (morphometrics) and deep features (CNN) methods to conduct morphological visualization analysis of tuna and perform principal component analysis and identification performance analysis, which can effectively study the interspecific differences and biodiversity of morphological characters of tuna species of the same genus.
Among different machine learning algorithms to identify tuna, EFT features revealed the best identification performance in KNN, with an average identification accuracy rate of 88 percent. Deep features showed the best performance with an average of 90 percent. The data obtained indicate that the analysis of morphological outlines supports the existence of three morphotypes (diversity of interspecific differences) within the studied tuna species.
This study verifies the interspecific differences in the morphological characteristics of different tuna species through different performance analytic methods. Automated identification using different machine learning algorithms is helpful to further understand the morphological specificity of tuna. The comparative analysis of different morphological characteristics shows that the morphological data of deep features can be used to analyze the biodiversity of tuna. This method will promote the development of tuna fishery monitoring and provide a feasible strategy for fish biodiversity research in the future.
Now that you've reached the end of the article ...
… please consider supporting GSA’s mission to advance responsible seafood practices through education, advocacy and third-party assurances. The Advocate aims to document the evolution of responsible seafood practices and share the expansive knowledge of our vast network of contributors.
By becoming a Global Seafood Alliance member, you’re ensuring that all of the pre-competitive work we do through member benefits, resources and events can continue. Individual membership costs just $50 a year.
Not a GSA member? Join us.
Author
-
Dr. Bilin Liu
Corresponding author
College of Marine Sciences, Shanghai Ocean University, Shanghai 201306, China
Tagged With
Related Posts
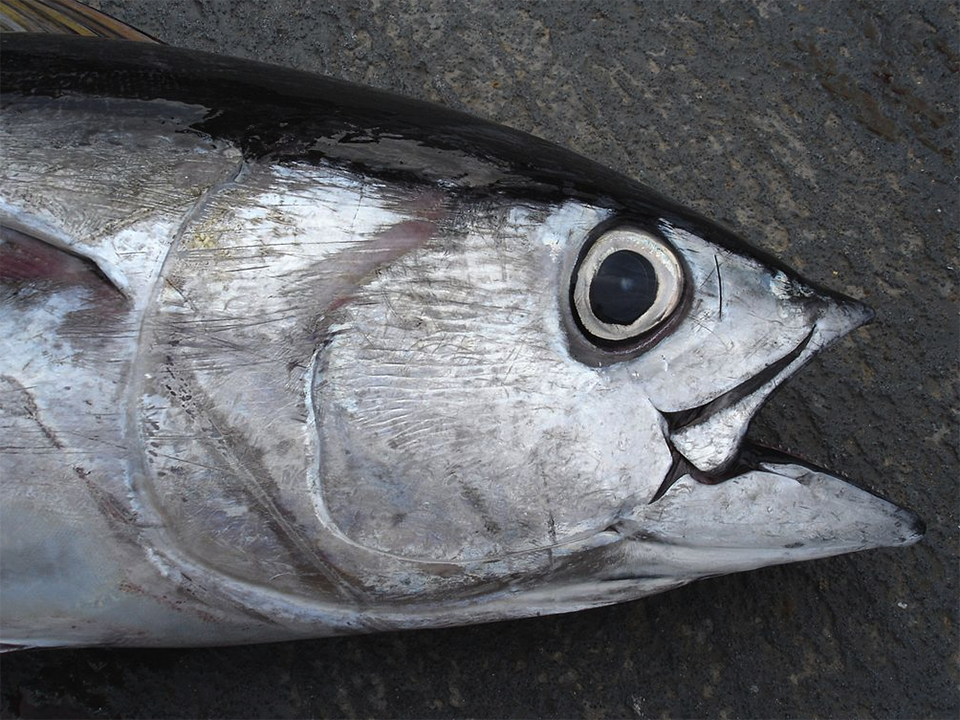
Fisheries
Fisheries in Focus: Marine protected areas don’t help tuna population, new paper shows
Using stock assessment and fishing data, researchers find that the Phoenix Islands Protected Area did not meaningfully increase the tuna population.
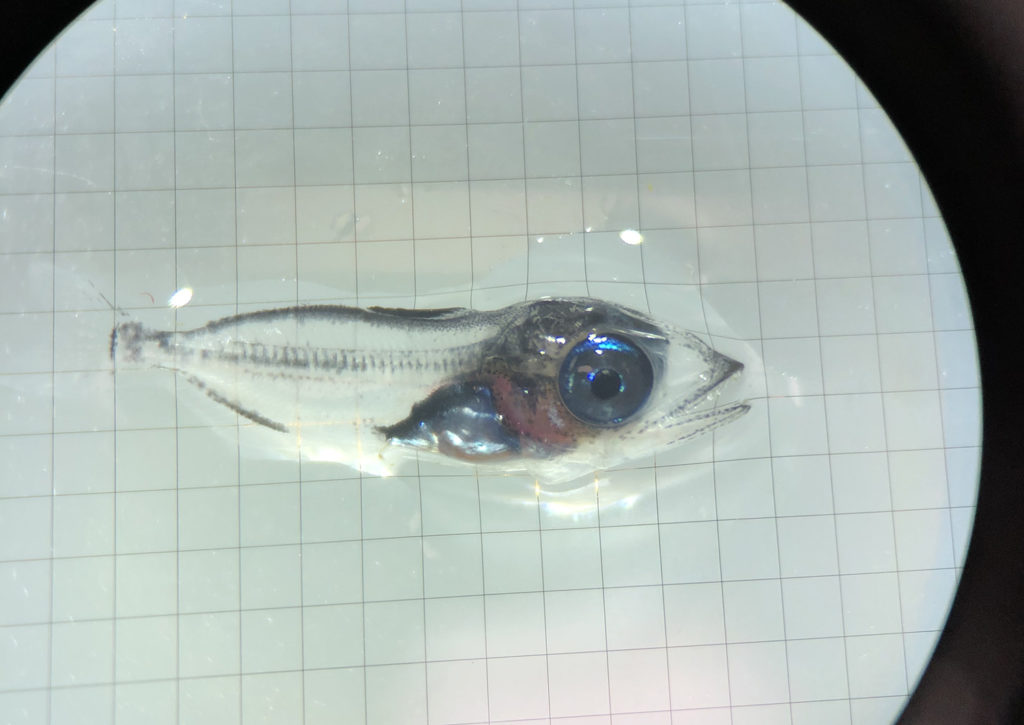
Innovation & Investment
‘Not convinced it can’t be done’: A look inside California’s new bluefin tuna hatchery
San Diego-based bluefin tuna hatchery and feed company Ichthus Unlimited aims to make tuna ranching a more sustainable and reliable option.
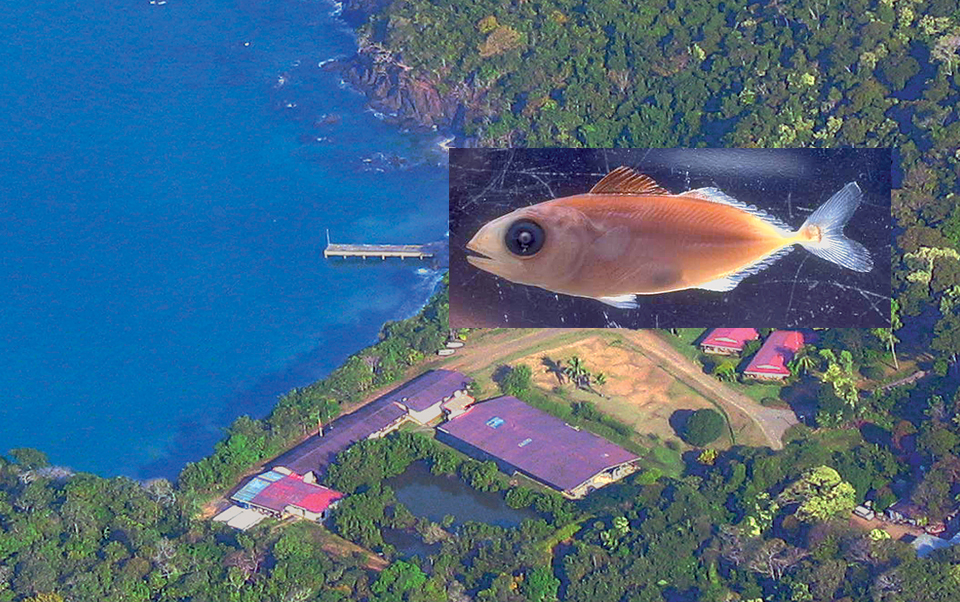
Health & Welfare
Achotines lab studies diets for larval, juvenile yellowfin tuna
Research at the Achotines Laboratory in Panama has been inconclusive involving probiotic treatments of first-feeding larval tuna and the rotifers they eat.
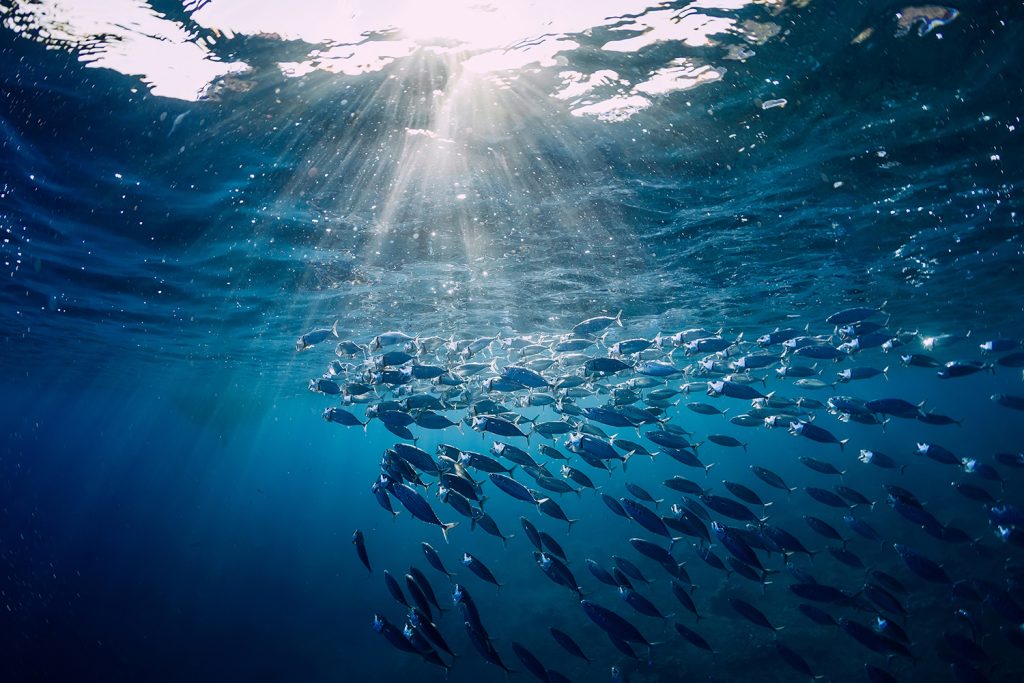
Fisheries
Fisheries in Focus: Tunas and billfishes are improving on the IUCN Red List – and it’s thanks to effective fisheries management
Sustainable Fisheries UW offers a rundown of the IUCN Red List and how tunas and billfishes benefit from strong fisheries management.